The Explosive History of Artificial Intelligence AI
The History of Artificial Intelligence: Key Events from 1900-2023
Critics, however, find fault with Deep Blue for winning merely by calculating all possible moves, rather than with cognitive intelligence. Learn of artificial intelligence (AI) are raising troubling concerns about its unintended consequences. Biometric protections, such as using your fingerprint or face to unlock your smartphone, become more common. Tesla
view citation[23]
and Ford [newline]view citation[24]
announce timelines for the development of fully autonomous vehicles.
In summary, the resurgence of interest in neural networks and connectionism in the 1980s and 1990s, driven by the backpropagation algorithm and advances in hardware, laid the foundation for the development of deep learning. These developments transformed the field of AI and continue to underpin many of the state-of-the-art AI systems we use today. From banking, technology, and healthcare to marketing and entertainment, AI has achieved what once seemed to be inconceivable. The future of AI is bright as it’s poised to steadily improve further and significantly change how we live and work.
The development of artificial intelligence since its beginnings
With dedicated mentoring sessions, you’ll know how to solve a real industry-aligned problem. You’ll learn various AI-based supervised and unsupervised techniques like Regression, Multinomial Naïve Bayes, SVM, Tree-based algorithms, NLP, etc. The project is the final step in the learning path and will help you to showcase your expertise to employers.
Foundation models, which are large language models trained on vast quantities of unlabeled data that can be adapted to a wide range of downstream tasks, began to be developed in 2018. The main disadvantage of foundation models is their requirement for enormous computational power and data, leading to high costs and potential accessibility issues for smaller organizations. Today’s artificial intelligence landscape is evolving with unprecedented speed. With a market that’s expected to grow to $2 trillion by 2030, AI is changing industries across the board — from eCommerce to healthcare and cybersecurity. While we can only speculate on what experts have in store, there are a few trends that’ll define the next decade. The explanatory film of the Plattform Lernende Systeme outlines different phases of technology development, milestones in AI applications as well as challenges that arise when using AI.
The history begins: the term ‘AI’ is coined
In this topic, we’ll embark on a voyage that traces the origins, milestones, challenges, and triumphs of AI development or in short we will uncover the brief history of AI. We spoke to him about his idea behind such an excellent app and his whole journey during the development process. MobileAppDaily had a word with Coyote Jackson, Director of Product Management, PubNub. We spoke to him about his journey in the global Data Stream Network and real-time infrastructure-as-a-service company.
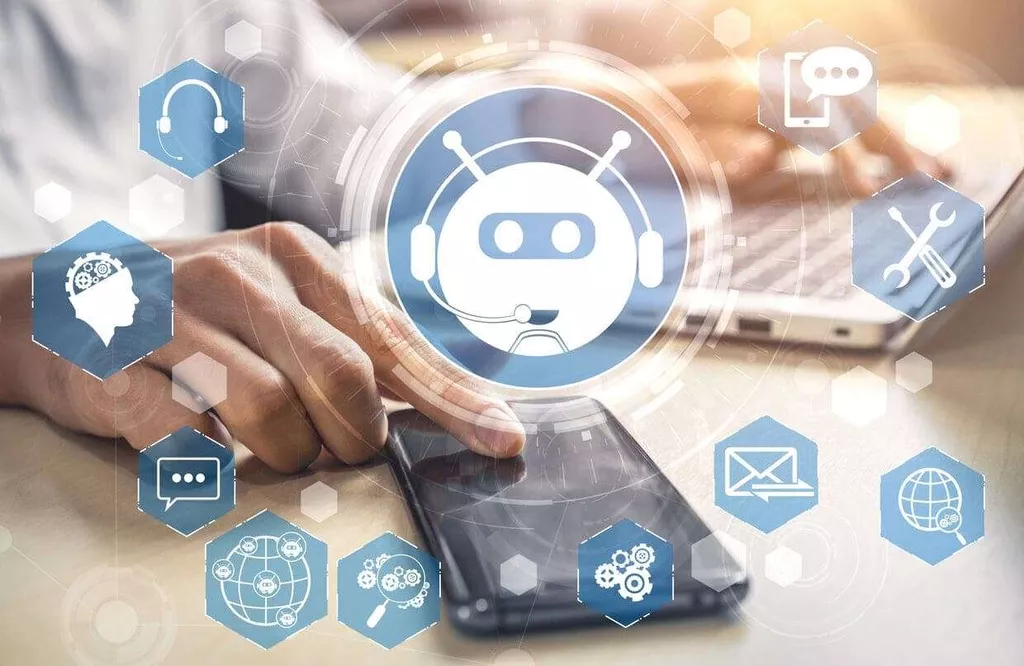
This development would rely on vastly more advanced technology than what we have today. Before the emergence of big data, AI was limited by the amount and quality of data that was available for training and testing machine learning algorithms. Another example is the ELIZA program, created by Joseph Weizenbaum, which was a natural language processing program that simulated a psychotherapist. In 1956, the Dartmouth Workshop marked the official birth of AI as a field of study. Researchers at Dartmouth College aimed to simulate human thought processes using computers.
The Turing Test’s Introduction
In 1956, scientists gathered together at the Dartmouth conference to discuss what the next few years of artificial intelligence would look like. The German-American computer scientist Joseph Weizenbaum of the Massachusetts Institute of Technology invents a computer program that communicates with humans. ‘ELIZA’ uses scripts to simulate various conversation partners such as a psychotherapist. Weizenbaum is surprised at the simplicity of the means required for ELIZA to create the illusion of a human conversation partner. In doing so, he lays the foundation for what we call artificial intelligence today. AI is a more recent outgrowth of the information technology revolution that has transformed society.
CNNs matured from being a promising concept to an indispensable tool, not just for image classification or object detection but for the broader canvas of AI applications. The previous chart showed the rapid advances in the perceptive abilities of artificial intelligence. These machines do not have any memory or data to work with, specializing in just one field of work.
Concept of AI in the Real World – The Beginning of Artificial Intelligence!
As a result of these and other limitations, classical AI experienced several public failures. These failures included deficiencies in machine-based language translation, limitations of the first neural-networks (known as perceptrons), and the highly critical Lighthill Report. They are not trained for specific tasks but rather provide a versatile base that can be fine-tuned for various applications. This approach has led to more efficient and effective AI systems, capable of performing tasks ranging from language translation to content creation with remarkable proficiency. The main disadvantage of machine learning is its dependency on large, high-quality datasets.
- But these systems were still limited by the fact that they relied on pre-defined rules and were not capable of learning from data.
- Research and development of other AI projects over time have contributed to numerous advancements that we now use in our everyday lives.
- To lay the foundation of that idea, we mentioned some tales from mythology and movies in the beginning.
- This period of stagnation occurred after a decade of significant progress in AI research and development from 1974 to 1993.
A. Simon (RAND Corporation) develop the General Problem Solver (GPS-I), a computer program intended to work as a universal problem solver machine. AI was now able to create images, write text, and recognize and mimic speech patterns. The First AI Winter ended with the promising introduction of “Expert Systems,” which were developed and quickly adopted by large competitive corporations all around the world. The primary focus of AI research was now on the theme of accumulating knowledge from various experts, and sharing that knowledge with its users. Remember, in the world of business, knowledge is not just power; it’s the engine of transformation.
DATAVERSITY Resources
Later on in the year 1965, the first expert system was designed by Edward Feigenbaum and Joshua Lederberg known by the name DENDRAL (Dendritic Algorithm). This expert system had the capability of mapping the structure of molecules available in different compounds. The data was compared with the existing data to determine which one existed and which one didn’t. It helped in automating the task of detecting new compounds for organic chemists. This was another huge breakthrough in the evolution of artificial intelligence.
- These limitations included limited computing power available in the 1950s and 60s, the exponential growth of computational complexity for non-trivial AI problems, and the limitations of purely symbolic computation.
- Elon Musk, Steve Wozniak, and others call for a six-month pause in training more advanced AI systems.
- Around 1985, companies were spending over $1 billion each year on the technology; but by the early 1990s, these systems had proven expensive to maintain, difficult to scale, and limited in scope, and interest died down.
- Discover how it took off, from Alan Turing’s test, to the advent of ChatGPT.
- At that time high-level computer languages such as FORTRAN, LISP, or COBOL were invented.
The reason was simple, the AI technology was limited by the technology of its time. As per Moore’s Law, the number of transistors that can be fixed in a chip doubles every year. This law has been true since the 1960s making it possible for us to have supercomputers like Frontier. A supercomputer capable of processing 1.194 quintillion floating point operations per second (FLOPS).
The Start of the AI Renaissance and Machine Learning: 1990s-2000s
This happened in part because many of the AI projects that had been developed during the AI boom were failing to deliver on their promises. The AI research community was becoming increasingly disillusioned with the lack of progress in the field. This led to funding cuts, and many AI researchers were forced to abandon their projects and leave the field altogether. As we spoke about earlier, the 1950s was a momentous decade for the AI community due to the creation and popularisation of the Perceptron artificial neural network.
How to get ready for rise of AI: Look at the history of disruption – Business Insider
How to get ready for rise of AI: Look at the history of disruption.
Posted: Thu, 31 Aug 2023 07:00:00 GMT [source]
The Alan Turing Institute, the UK’s national institute for data science, opens. Headquartered in the British Library, Oxford is one of five founding universities. Honda’s ASIMO (Advanced Step in Innovative Mobility robot), an artificially intelligent humanoid robot, is unveiled. World chess champion and grand master Gary Kasparov is defeated by IBM’s Deep Blue, a chess playing computer program. The rise of the internet in the 1990s leads to an explosion of digital data, providing the raw material for more sophisticated AI algorithms. Wabot-2 is revealed at Waseda University in Japan, a musician humanoid robot.
Overall, expert systems were a significant milestone in the history of AI, as they demonstrated the practical applications of AI technologies and paved the way for further advancements in the field. This research led to the development of new programming languages and tools, such as LISP and Prolog, that were specifically designed for AI applications. These new tools made it easier for researchers to experiment with new AI techniques and to develop more sophisticated AI systems. The participants set out a vision for AI, which included the creation of intelligent machines that could reason, learn, and communicate like human beings. Early examples of models, like GPT-3, BERT, or DALL-E 2, have shown what’s possible. The future is models that are trained on a broad set of unlabeled data that can be used for different tasks, with minimal fine-tuning.
Read more about The History Of AI here.